I went back and looked at the projections at the start of 2023 and compared them to the actual results.
On average the Fangraphs projection missed by 9 games. The largest misses were:
Orioles - 24 games
A's - 20 games
White Sox 19 games
Royals 17 games
Mets 16 games
The R squared was REALLY not very predictive.
Baseball is very unpredictable, and we don't know who is going to win.
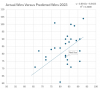
On average the Fangraphs projection missed by 9 games. The largest misses were:
Orioles - 24 games
A's - 20 games
White Sox 19 games
Royals 17 games
Mets 16 games
The R squared was REALLY not very predictive.
Baseball is very unpredictable, and we don't know who is going to win.
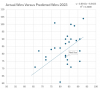